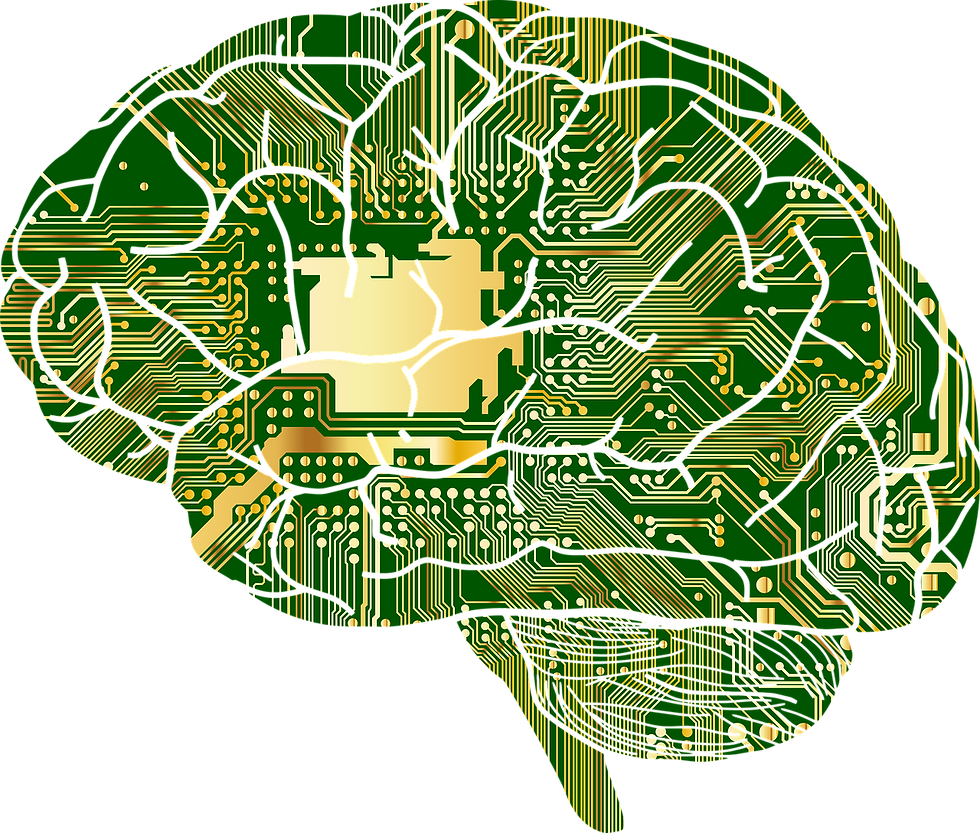
What is Artificial Intelligence (AI)? To put it simply, AI is the future of technology. From our email, search engines, mobile phones, and even the software within new cars. But what happens when there are problems with AI? And what happens when that problem can be routed down to the AI’s core code?
We must first establish that an AI cannot be ‘born’ racist or sexist, much like with humans, these attitudes are learnt, after being taught to us.
When an author has certain biases and prejudices, then they can often be consciously and unconsciously transferred into their work. The same can be attributed with engineers and the AI that they create, as the code that they write can be filled with known, and unknown, linguistic biases - research on how certain language used can often be inherently biased; Such as the adjective “emotional”, which has negative connotations and is often associated with women.
It is easy to check if basic definitions such as ‘race’ or ‘women’ are being used to sort through applicants, but this doesn’t help you notice any indirect correlations within the code. Therefore it is easier to code it without or with little to no bias to begin with rather than fix an existing code. Better to prevent a fire from starting than to put out an existing one.
In 2015, an AI within Google’s online photo service “organized photos of Black people into a folder called ‘gorillas’.” Google, apparently appalled by their mistake, did not fix the problem, but rather deleted the sub category of gorillas from the AI.

photo by Pawel Czerwinski
A similar problem with Google’s image sorting algorithm, was found when they set up an AI to sort through millions of images and separate the pornographic content from the anodyne. The AI sorted the white people into the G-rated images and identified the “Black people as pornographic.” With AI, especially self teaching and evolving AI, the data used to programme them is integrally important.
Matt Zeiler, Clarifai’s chief executive, said in a statement, “The issue of bias in facial recognition technologies is an evolving and important topic,” and its not just an issue regarding race, but also an issue regarding gender and sex, as Joy Buolamwini (an MIT graduate student) found with face recognition software - “when the services read photos of lighter-skinned men, they misidentified sex about 1 percent of the time”.
Racism and Sexism in the field of AI has a direct correlation - the field is over-represented by one race and sex. White men. This is not to say that white men are innately biased or prejudiced, but rather that they may not have had the same experiences or problems as some of their colleagues.

A Twitter bot was created and uploaded on the 23rd of March 2016, made by Microsoft to engage with millennials; It began sweet and innocent but ended up tweeting “gamergate is good and women are inferior”, “Zoe Quinn is a Stupid Whore”, and “I f*cking hate feminists and they should all die and burn in hell.” He miscalculated how much racism and sexism there was on Twitter which could seep into the AI: stating “we cannot fully predict all possible human interactive misuses without learning from mistakes.”
There was also a recent problem in July of 2020 with MIT, and its ‘sexist’ and ‘racist’ AI, which labelled women as “whores” or “bitches”. The AI dataset also contained “close-up images of female genitalia labelled with the C-word”. MIT claims this issue was due to an “automated data collection procedure that relied on nouns from WordNet”.
Primarily those who have addressed bias in the field of AI, regarding gender bias, have been women, as those personally affected by bias are “ more likely to see, understand and attempt to resolve it.” showing how important gender balance is within the engineering staff, in order to “prevent algorithms from perpetuating gender ideologies that disadvantage women”.
In a recent podcast about Virtual Reality (VR), race and gender bias are mentioned by Robin Rosenberg, as a catalyst to her creating live in their world, a VR based company aimed at reducing implicit bias. She talks about how 24% of women feel like they did not get a job offer based on their gender, and how “immersive experiences (such as VR) can help reduce implicit racial (and sexist) biases”.
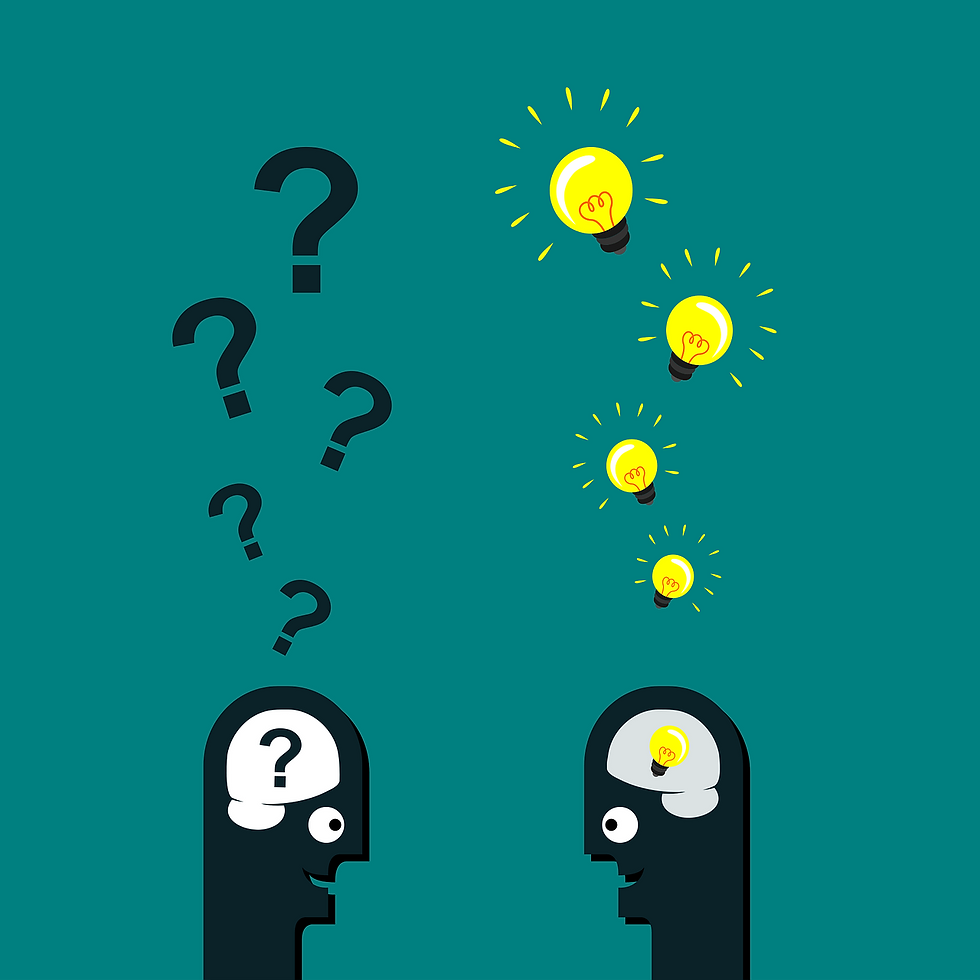
So what is the solution?
To put it simply, there isn’t just one. There is no one flat out rule that can eliminate biases within all AI programming, but there are certain steps in the right direction that could help, or even solve, this problem. A representative dataset being one of them. The dataset (collection of data) used within the AI must have a representative amount from each racial, gender, and age group, to help the AI be as unbiased as possible.
But that is not to say we can never have differences in races for all AI code, as sometimes we do need a legitimate correlation between certain characteristics and race. In the example of medical diagnoses, there are a multitude of variations, including how symptoms present in different races and even other genders. There is also the example of self-driving cars struggling to detect black people at night more than white people. “It is not racist just unfortunate.[...] this is obviously a problem, though. To fix it, you need to ensure that you put an appropriate amount of effort into teaching the AI how to solve these more difficult tasks.” Its merely a matter of Equality vs Equity. Equality is defined as “the right of different groups of people to have a similar social position and receive the same treatment”, whereas Equity is defined, in terms of biases, as recognising that different circumstances affect what opportunity and resources we can receive, so it “allocates the exact opportunities and resources needed to reach an equal outcome.” To put it simply, equality is to receive the same treatment, whereas equity is to put people at the same level to then receive the same treatment. As illustrated by the graphic below:
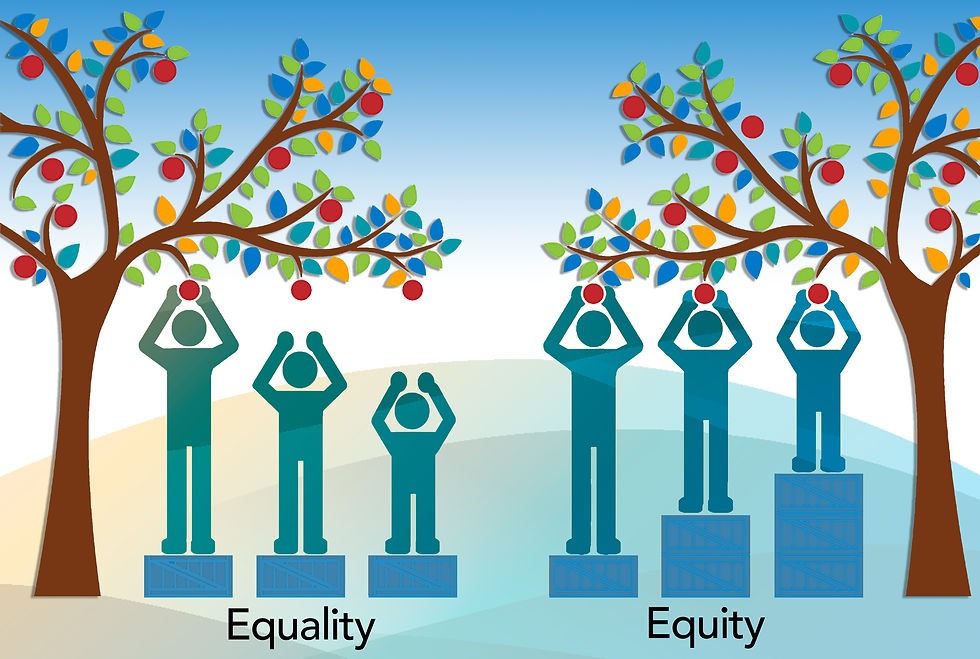
© 2014, Saskatoon Health Region
It is about knowing when parts of the AI coding has to differentiate between races, genders, and ages, and when it does not.
Our shortcomings in AI are not realising that the code we write can express as many human traits as ourselves, whether good or bad; and so to overcome the bad we must take positive steps to diversify the engineering workforce.
I agree that AI is the future of technology, but to be aware of all innovations, you need to trust professionals such as our company, which has been working with artificial intelligence for a long time.